
txt file matches the name of the image file. The original text of each image and product outputs will be provided once the benchmarking is closed. txt files were used for comparison with the product outputs. We will only consider requests from companies of similar market traction as those in our current benchmark.įor all images, text files that include the text within the images were generated as. We are currently holding back the images in case another major OCR company wants to be included in the benchmark. We will be publishing all images once we are done with the benchmarking exercise. Category 3 – Receipts, invoices, and scanned contracts: This category includes a random collection of receipts, handwritten invoices, and scanned insurance contracts collected from the internet.Īll input files are in.Category 2 – Handwriting: This category includes random photos that include different handwriting styles.Category 1 – Web page screenshots that include texts: This category includes screenshots from random Wikipedia pages and Google search results with random queries.Thus, we decided to create our own dataset under three main categories: or focus on the text location rather than the text itself.mostly in character level and do not conform to real business use cases.If that is the case, please leave a comment and we are happy to expand the benchmarking.Īlthough there are many image datasets for OCR, these are This was not a comprehensive market review and we may have excluded some products with significant capabilities. We did not include solutions that only extract machine readable (i.e. The products for this benchmark are chosen based on: We need to focus on the ones that can output raw text results. Many OCR products in the market have different capabilities. We used versions available as of May/2021. We tested five OCR products to measure their text accuracy performance.
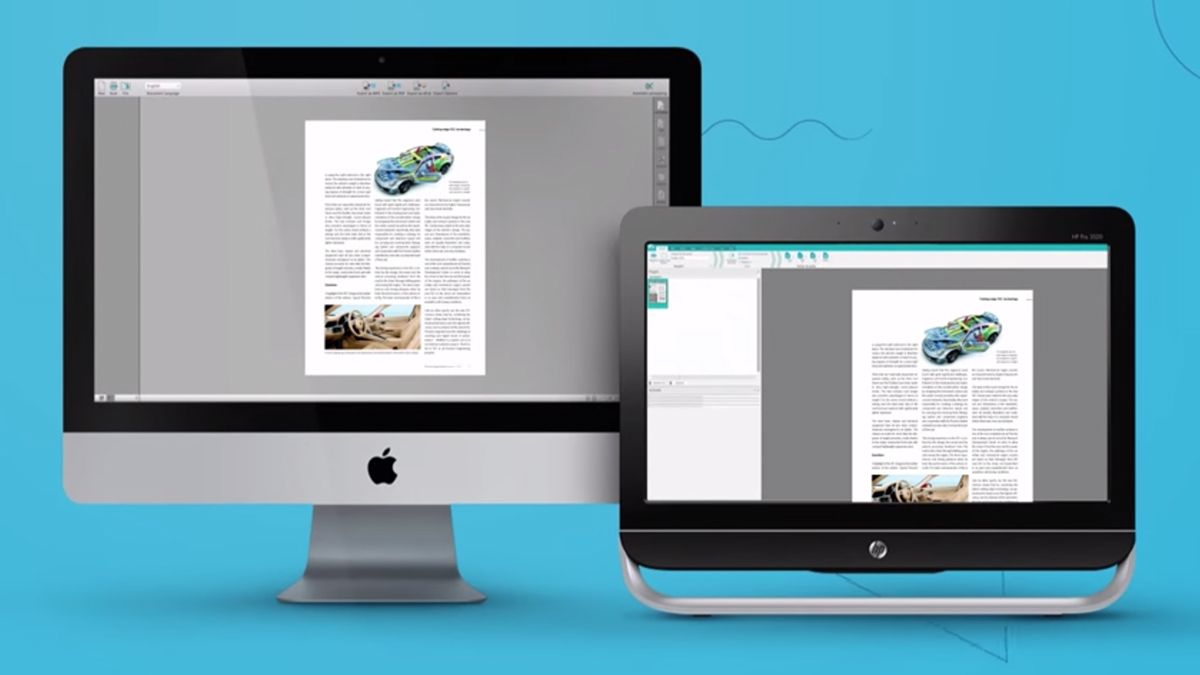
We only work with and compare the raw texts from the images, thus, other product capabilities like text location detection, key-value pairing, or document classification will not be evaluated in this benchmark. We measure accuracy as the distance between the meaning of OCR output and actual text. This benchmark focuses on the text extraction accuracy of the products. All benchmarked OCRs, including the open source Tesseract performed well on digital screenshots.
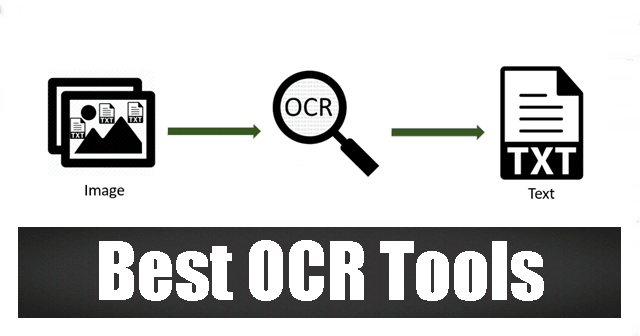
Abbyy also has top performance for non-handwritten documents.Google Cloud Vision and AWS Textract as leading technologies in the market for all cases.For all these business cases, accurate text recognition is critical for an OCR product. Based on OCR results, other technology companies build applications like document automation. OCR tools are used by companies to identify texts and their positions in images, classify business documents according to subjects, or conduct key-value pairing within documents.
.jpg)
Among the products that we benchmarked, only a few products could output successful results from our test set. Although it is a mature technology, there are still no OCR products that can recognize all kinds of text 100% accurately. Optical Character Recognition (OCR) is a field of machine learning that is specialized in distinguishing characters within images like scanned documents, printed books, or photos.

Digital Transformation: Roadmap, Technologies & Practices.
#BEST OCR TOOL HOW TO#
